Artificial Intelligence (AI) refers to computer processes that display human-like intelligence, such as thinking, learning, perceiving, and decision-making. This is a broad term that encompasses various applications. In fact, AI is already a part of our everyday lives, often without our awareness. For instance, AI algorithms are used to scan our medical information, predict disease diagnoses, and identify individuals at risk of suicide to prevent untimely deaths (Morales et al., 2017).
Artificial Intelligence (AI) has three main applications in mental health today. Firstly, AI is used to assist doctors in making a psychiatric diagnosis by analyzing the responses to a short interview, self-report questionnaires, and the patient’s response to previous interventions (Kravets et al., 2017). Secondly, natural language processing models are utilized to analyze the content of therapeutic sessions, providing feedback to therapists to improve their techniques. Finally, programs and mobile applications offer computed therapy based on established protocols, such as Cognitive Behavioral Therapy and Mindfulness-Based Stress Reduction (Fitzpatrick et al., 2017). Some of these applications are commercially available, while others are still in the research phase.
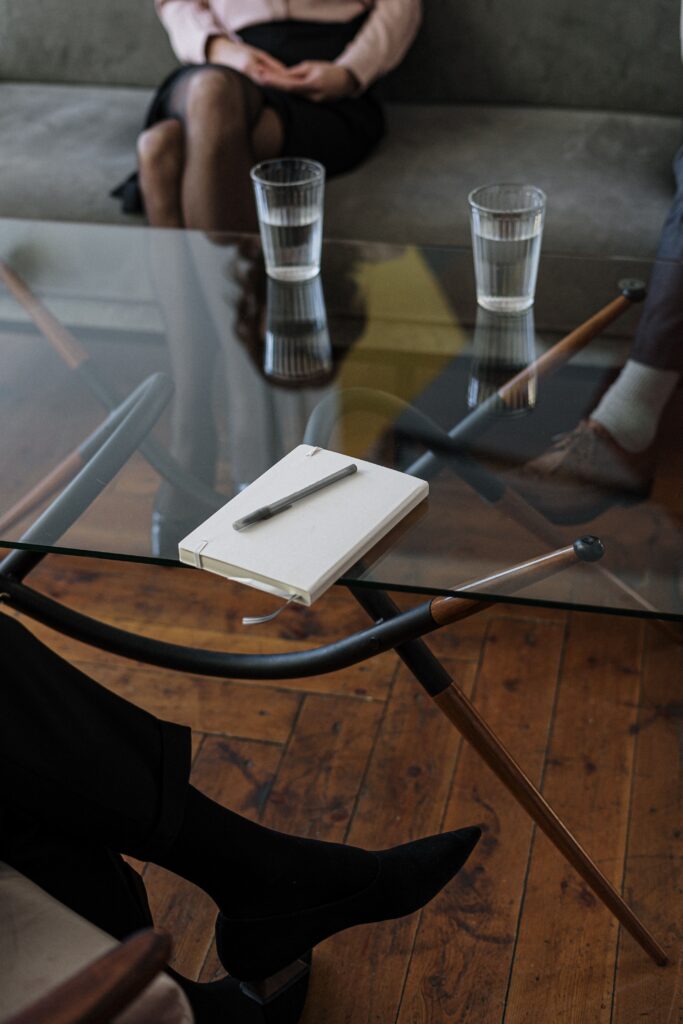
Large language models (LLMs), like ChatGPT and Bard, are worth mentioning, as they have become widely used and noticed by everyone. But when it comes to therapy, or automated therapists, things get a bit tricky. Therapeutic applications and computer programs are based on a relatively “old” technology known as intent recognition. Intent recognition is the process of identifying the purpose or goal behind a piece of text or speech. In the context of language models, intent recognition involves analyzing the input text to determine the specific action or information that the user is trying to convey. This is a crucial component of natural language understanding, as it allows the model to interpret and respond to user queries or commands accurately. Intent recognition is often used in applications such as chatbots, virtual assistants, and customer service platforms, where understanding user intent is essential for providing effective and efficient interactions.
In therapeutic interventions with a specific purpose, such as identifying maladaptive thoughts or teaching patients to monitor their emotions, intent recognition can be relatively straightforward. I personally had some insightful conversations with Woebot (Fitzpatrick et al., 2017) during a stressful period in my life. Woebot helped me identify the thoughts, behaviors, and emotions that contributed to my distress and provided some relief from my anxiety. However, it raises the question of whether I could trust Woebot as much as I trust my therapist. Moreover, it can be difficult to shake the feeling that I am not speaking to a human being who may have experienced something similar but rather to a computer program consisting of lines of zeros and ones, delivering pre-recorded responses that aren’t always on the dot.
We are working on the SOCRATES project at the Advanced Reality Lab at Reichman University to develop an AI system that can mimic human-like responses. Rather than simply providing pre-recorded responses based on intent recognition, we aim to use state-of-the-art language models like LLMs to create a more sophisticated system. However, to do so, we need to train the model using data from actual therapy sessions so that it can learn how to respond like a therapist. Despite having access to vast amounts of internet data, currently, it seems that these language models may not fully comprehend the intricacies of therapy and how a therapist would respond. Given their performance on other tasks, this is not unexpected. Despite our best efforts to train the model using therapy data and prompts to encourage therapist-like responses, the model’s responses remain inconsistent at times, ranging from outstanding and insightful to unconstructive. Due to the sensitive nature of therapy, we must exercise extra caution when developing these models for this type of purpose.
While the mission we have undertaken is undoubtedly challenging, we are confident that we will be able to push forward and develop more advanced solutions using the latest technology. Our ultimate goal is to enhance our lives and the lives of those around us.
About the author
Momi Zisquit is a clinical psychologist and a Ph.D. candidate at the ARL lab run by Prof. Friedman at Reichman University in Israel. Her Ph.D. thesis regards the application of virtual reality to the field of suicide prevention. She is collaborating on the SOCRATES project, contributing to the development of the AI model as well as running studies with ConVRSelf.